Exploring automated output checking with OpenSAFELY
- Posted:
- Written by:
-
- Mike Thompson
- Categories:
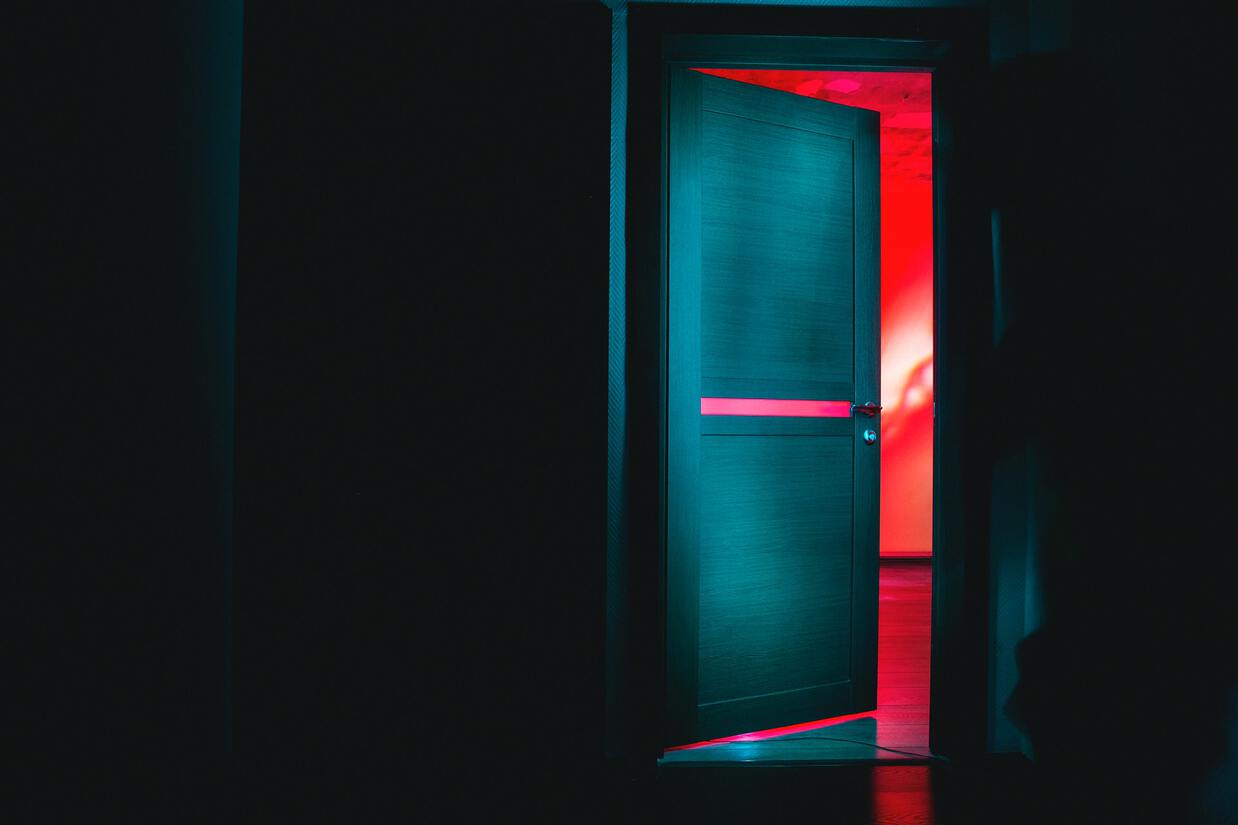
This is a guest blog from the team at Cantabular, who have been exploring how their technology might fit into the OpenSAFELY ecosystem.
Over the summer months, we had a few conversations with the interesting folk at the University of Oxford Bennett Institute who have been building OpenSAFELY—an open source, transparent platform for secure analysis of electronic health records—to see if we could integrate our automated output checking capability into their systems, to help speed the process of release of research outputs.
A potential collaboration
OpenSAFELY’s platform significantly improves data security and transparency around the use of electronic health records (EHR) data in epidemiological research. Prior to publication, the team uses experienced researchers to manually double-check outputs from studies to ensure that any potentially disclosive outputs are suppressed or otherwise made safe.
Cantabular includes a component, called Cantabular Audit, that uses rules written in a domain specific language to automatically evaluate cross-tabular outputs for specific disclosure risks such as identity disclosure, attribute disclosure and sparsity.
We were interested in understanding whether our automated checks could be used by OpenSAFELY to reduce the amount of manual checking needed, particularly as the quantity of research being carried out through the platform increases.
Technical proof of concept
To explore the technical feasibility of a collaboration, we made a quick proof of concept and a video of it in action:
The demonstration above shows the possibility of an integration, but raises a number of further questions:
- Workflow: what’s the best place for this kind of audit to sit within a researcher’s workflow? A final check of outputs at the end or an analysis of the source dataset at the start? It’s hard to ask researchers to make a change to existing working practices that may slow down their work without a clear incentive.
- Inputs: is there a more realistic way to characterise the inputs we’d have to handle? EHR data can be hard to work with because of the multiple sources, challenges in constructing variables, and constant change as patients move in and out of the data.
- Disclosure checks: what are the biggest risks inherent in the data and what checks are researchers and data controllers currently performing manually? Is low number suppression enough? How might you take into account cumulative risks over time and between different studies? How do you effectively check outputs from statistical analyses such as regressions, box plots or survival analyses?
- Outputs: What kind of output would be most useful for researchers to get from an audit like this? Should the software be doing suppression or perturbation itself, or merely flagging risks?
To explore these questions we arranged some further conversations with a couple of willing and generous researchers from the OpenSAFELY team.
Exploring options for privacy protection
The more detailed conversations with the OpenSAFELY team helped us to better understand their needs, their current processes, and opportunities and challenges around automated privacy protections.
While epidemiological studies such as those being produced by the OpenSAFELY team often include cross-tabulations to characterise the population, many other kinds of outputs are produced, each of which can have its own disclosure considerations. (For an excellent overview of different outputs and their disclosure considerations, have a look at the Secure Data Access Professionals’ Handbook on Statistical Disclosure Control for Outputs.)
In a situation like this, what are the different options available for privacy protection? A straightforward way of thinking about them is the location in the research process where the privacy protections are applied:
- At the source: a dataset can be altered at its source using techniques such as k-anonymisation or differential privacy to render it safe for subsequent analysis. These approaches inevitably lead to a loss in utility through less resolution in variable categories or less accuracy in metrics or observations which may be unacceptable in some situations. The advantage, however, is in minimising the disruption to the rest of the research process, giving more freedom to subsequent analysis and giving strong guarantees about the level of privacy protection.
- In the method: an alternative to altering the data at the source, is to provide researchers with specific analytical methods where the disclosure protections are built in, such that the output produced by the method is guaranteed to be non-disclosive, within given parameters. For example, a scatter plot or box plot method could automatically suppress outliers to avoid attribution of individual observations. For more on this, see Privacy preserving data visualizations (2021) by Avraam et al.
- At the end: outputs can still be checked at the end of an analysis process, either through automated or manual checks, to identify potential disclosure problems. For automated checks to work, however, it is inevitable that outputs need to be supplied in standard formats and the rejection of an output implies the need for a further iteration by the researcher to make it acceptable.
Of course, different approaches can be used in combination, such as in Norway’s microdata.no project (PDF), or used individually in different circumstances. For example, for frequently produced analyses of time series data which involve reusing the same methods repeatedly, automating protections within the methods themselves may be ideal and remove the need for any further checks. A unique piece of one-off analysis, however, may inevitably require manual checks.
We’ve enjoyed our little summer sojourn into the world of electronic health record data, and we’ll be keeping our eyes open for other potential research projects to collaborate on. In the meantime, OpenSAFELY have an excellent team who are exploring these issues themselves and building their own methods for more automatic protection of the data.